Multispectral Analysis of Multiparameter MRI

Multiparameter MRI phenotypes of normal tissues and intratumoral habitats in pancreatic ductal adenocarcinoma
Tissue type maps of normal brain and recurrent glioblastoma at various times post-initiation of treatment (C1D1)
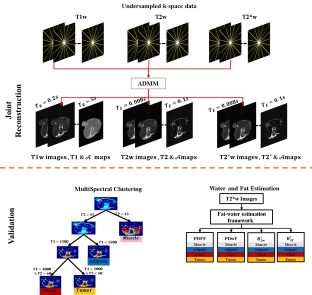
Joint reconstruction of undersampled co-registered mpMRI raw data to accelerate the acquisition of intratumoral habitat maps.